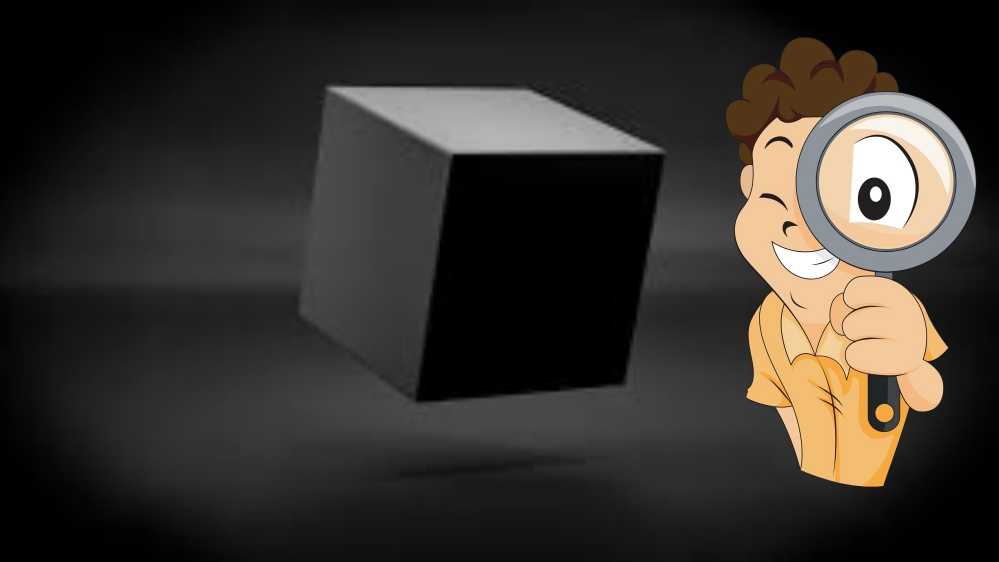
Introduction to Black Box AI:
Black Box Artificial Intelligence means AI systems where we can’t see how they work inside. This means users can’t understand why the AI makes the decisions it does. Even though these systems can be right, we can’t see why they choose certain things. This is a problem, especially in important areas like healthcare, finance, or law enforcement.
Importance of Understanding Black Box AI
Accountability:
- When we don’t know how a system makes decisions, it’s hard to blame it for mistakes or unfair results.
Fairness:
- AI systems that are hard to see inside might keep unfairness going by treating some people .
Security:
- Bad people can take advantage of problems in Black Box AI systems because we can’t look at them to find flaws.
Trust:
- People need to believe that AI systems will make good choices. When we can see how they work, it helps us trust them more.
Challenges and Approaches to Addressing Black Box AI
Explainable AI:
- Scientists are working on ways to make AI easier to understand, so people can see why it makes certain decisions.
Regulatory Frameworks:
- Governments and organizations are creating rules to control the use of AI in risky situations, aiming to prevent problems.
Transparent Development Practices:
- Following rules and good ways of making AI from start to finish can make it easier to see inside AI systems and make sure they’re not mysterious.
In conclusion, understanding Black Box AI is important to make sure AI systems are safe, fair, and work well. While progress is happening to deal with the problems of Black Box AI, we still need to keep working on making AI .
Understanding Black Box AI:
Black Box AI means AI systems where we can’t see how they work inside. This makes it hard to understand why they make certain decisions. Even though they can be right, not knowing how they decide things raises worries about whether they’re fair and accountable. Researchers are trying to make AI that’s easier to understand, called Explainable AI.
The black box issue is important for ethics and safety, especially in important areas like medicine, money, and law. While black box models are good for figuring out data, we need to be careful and consider other options, like white-box AI. When accuracy and clear explanations are important.
Challenges of Black Box AI:
The black box problem in AI is when it’s hard to know how AI systems and machine learning models make their decisions, especially with deep learning methods like neural networks. This makes things tricky because we can’t see inside and understand how they work.
Lack of Accountability:
- When we can’t understand how AI works on the inside, it’s hard to blame it for mistakes or bad results.
Bias Mitigation:
- AI can pick up and make existing biases worse. Without seeing how it works, it’s tough to find and fix these biases.
Regulation Compliance:
- Opaque AI systems make it hard for regulators to make sure that rules and laws are being followed.
To help with these issues, people are working on making AI systems easier to understand. They’re doing this through something called “Explainable AI” (XAI). XAI tries to show how AI systems make decisions, which helps people trust them more and hold them accountable. Also, sharing AI models can make it easier for everyone to see how they work and work together better in the AI community.
Even though people are trying to solve these problems. The black box issue is still a big worry when it comes to using AI in areas like healthcare, money, and catching criminals.
Implications for Society:
The implications of Black Box AI on society are significant, touching on privacy, ethics, and its impact on jobs. Black Box AI means artificial intelligence systems where the user can’t see how they work inside. This lack of transparency raises concerns about privacy, ethics, and job impact.
Privacy
Black Box AI’s secrecy raises worries about privacy. It can make choices about people without explaining why. For example, in healthcare or loan decisions, people might not know why AI made certain choices. Making them wonder about their privacy and if the decisions are fair.
Ethics
Using Black Box AI can bring up ethical problems because it might pick up on unfair biases from the real world. For instance, AI has shown biases based on race in decision-making. Since we can’t see how it works or hold it accountable, it might make unfair decisions in things like job interviews, loans, or medical care, raising ethical concerns about fairness and accountability.
Impact on Jobs
Using Black Box AI in important areas like money and catching criminals can affect jobs. Because people lack visibility into decision-making, it may cause concern about job loss or fairness in hiring processes. For instance, if AI decides who gets a job interview without explaining why, it can make people question if the hiring process is fair, affecting job opportunities.
To tackle these problems, people are working on making “explainable AI” to make AI systems easier to understand and hold accountable. But, we still need to figure out how to deal with these issues and talk about the role of AI in society to understand the risks and benefits of Black Box AI.
Black Box AI has big effects on society, like privacy, ethics, and jobs. People worry about fairness, accountability, and biases in AI because the decision-making process isn’t visible. This shows that we need to keep talking and working on these problems to make things better.
Ethical Considerations:
Black Box AI means AI systems that people can’t understand inside. This can cause worries about fairness, discrimination, and unexpected results. For example, unfair or unexplained outcomes can lead to unfair treatment, keep inequalities going, and make it hard to hold organizations accountable for using AI.
To deal with these worries, experts suggest different ways to make AI clearer. This includes Explainable AI (XAI), fairness-aware machine learning, checking and reporting, rules, and involving people affected. But it’s important to know that making ethical codes is a way to try to control things, and it might not solve all the problems.
The lack of transparency in AI can also raise questions about who’s responsible, who knows best, patient freedom, and trust. Since AI is becoming more important in making decisions, it’s important to make sure it’s clear and ethical.
Regulatory Frameworks:
There are rules and suggestions for Black Box AI to make it clearer and more responsible. In Europe, a group suggested seven things for trustworthy AI, like having humans in charge and making sure it’s strong and clear. In the US, Deloitte recommends that companies check all their AI tools and make a plan to manage risks and make rules. They also suggest keeping an eye on black box algorithms, being open about how AI works, and checking it .
In a recent article, Yavar Bathaee talks about why Black Box AI is a problem. He describes AI, especially deep neural networks, as complicated, and people struggle to see patterns in it. Bathaee doesn’t think having rules for transparency will fix it, because AI will keep getting more complicated. Instead, he suggests a bigger solution to deal with the problems with why things happen.
Mitigating Bias:
To reduce bias in Black Box AI, experts suggest several important strategies:
Audit Algorithms:
- Check algorithms for bias, especially in important areas.
Use Diverse Training Data:
- Make sure datasets represent different types of people to avoid bias.
Involve Humans:
- Have people help AI systems to reduce bias by giving explanations and insights.
Explainable Techniques:
- Use methods that show how AI makes decisions to find and fix bias.
Red Teams and Internal Reviews:
- Set up groups to challenge assumptions and find bias in AI.
Continuous Feedback Loops:
- Keep checking AI results to spot any unfair impacts.
Collaborative Work Teams:
- Bring together experts from different areas to find and fix bias.
Regular Updates:
- Keep algorithms up-to-date with changes in society to avoid new biases.
Public Policy Support:
- Encourage laws and rules that make sure AI is fair and ethical.
Research Investment:
- Support studies to understand bias in AI better and find ways to fix it.
Diversify the Field:
- Encourage a diverse range of people to work in AI to ensure they notice and address bias.
Advancements in Explainable AI:
New improvements in Explainable Artificial Intelligence (XAI) aim to make AI systems easier to understand and trust in different areas. Some important developments include:
Perceived Explainability:
- Studies show that Explainable Artificial Intelligence (XAI) makes AI seem more understandable than AI without XAI. This leads to more trust and acceptance.
Techniques:
- Various XAI techniques help us understand how AI makes decisions, covering text, images, audio, and videos.
Critical Applications:
- XAI is very important in areas like defense, healthcare, law enforcement, and self-driving cars. Here, it’s crucial for AI to be clear and accountable.
Future Directions:
- People are still figuring out ways to make XAI better, especially in tricky areas like medicine.
Toolkits and Taxonomies:
- People are making tools and categories to help researchers and experts choose the right XAI techniques for their needs.
These improvements show how XAI boosts trust in AI and encourages more industries to use it.
Real-world Examples:
Positive:
Scientists are studying modern machine-learning models, like neural networks, using explanation methods to understand how they make decisions. For example, MIT made ExSum, a system that tests rules made from explanations to learn more about how models work.
Doctors use deep-learning models in medical imaging, such as detecting COVID-19 with chest X-rays. Even though they work well, researchers are still figuring out the problems with their “black-box” nature. They want to make them clearer and easier to understand.
Negative:
Criminal justice and banking systems use biased data, denying loans or labeling people of color as repeat offenders.
Mistakes in facial recognition algorithms can hurt people’s feelings and worsen social problems by getting people’s identities wrong.
Imbalanced training data in medical facilities can create bias in deep-learning models, making them less reliable in their performance.
These examples stress fixing the black-box issue in AI, especially in critical decision-making areas. This is to ensure fairness, safety, and accountability for everyone involved.
The Future of Black Box AI:
Research and technology in Black Box AI aim to make AI clearer. Explainable AI (XAI) and open-source methods help build trust.
XAI reveals how AI works, building trust, especially in healthcare and finance. Open-source AI models promote collaboration and transparency.
Regulations push for more transparency and responsibility in AI. Guidelines encourage safe AI development and use.
In short, Black Box AI is becoming clearer with XAI and open-source methods, increasing trust and reducing risks.